Introducing Sentius
“Sentio” (Latin): “to feel, perceive, or think”.
George Bernard Show once said, “reasonable man adapts to the world. The unreasonable man refuses to adapt to the world. Therefore, all progress depends on the unreasonable men.”
From Lever to AI Agents and Agentic Organizations
Humanity thrives by both adapting to the world and reshaping it to suit its needs. From levers to division of labor, we’ve invented tools and methods to bend reality to our will. Today, we stand at the cusp of a new era with Generative and Autonomous AI—an emerging lever that promises to redefine how we solve problems and build our future.
At Sentius, we believe this new AI lever combines two powerful human-inspired methods of understanding the world. The first is analytical, grounded in logic and reductionism—our traditional approach to science and engineering. The second is model-free, intuitive pattern recognition—a skill humans rely on to effortlessly distinguish an apple from a nectarine. While analytical models give us structured, rule-based reasoning, model-free methods help us adapt to the unexpected, much like our own intuitive minds.
Modern AI, especially deep learning, excels at the model-free approach, learning from experience rather than rigid rules. It emulates what psychologists Daniel Kahneman and Amos Tversky call our “System 1” thinking: fast, intuitive, and pattern driven. Combined with the logical rigor of “System 2” reasoning, this powerful blend offers a glimpse of true Artificial General Intelligence (AGI)—a lever that can upgrade entire organizations to the next level.
At Sentius, our mission is to harness these capabilities to create “agentic organizations”: enterprises augmented by AI agents that reason, understand, and act with unprecedented agility and accuracy. We envision a future where businesses adapt swiftly, scale effortlessly, and thrive in ever-changing landscapes. Our work today is about building that future—one where AGI is the lever that reshapes our world, ensuring progress continues to depend on the “unreasonable” thinkers who dare to push humanity forward.
Introducing Sentius Teach & Repeat Platform
Today, we’re proud to announce our platform for upgrading organizations to the “agentic” form: Sentius Teach & Repeat Platform. It is designed to enable process leaders in the companies to create new automations at scale, by doing them from scratch automatically, or by showing manually executed steps to the system for it to learn them, and by giving tools to bring changes to the workflows when needed.
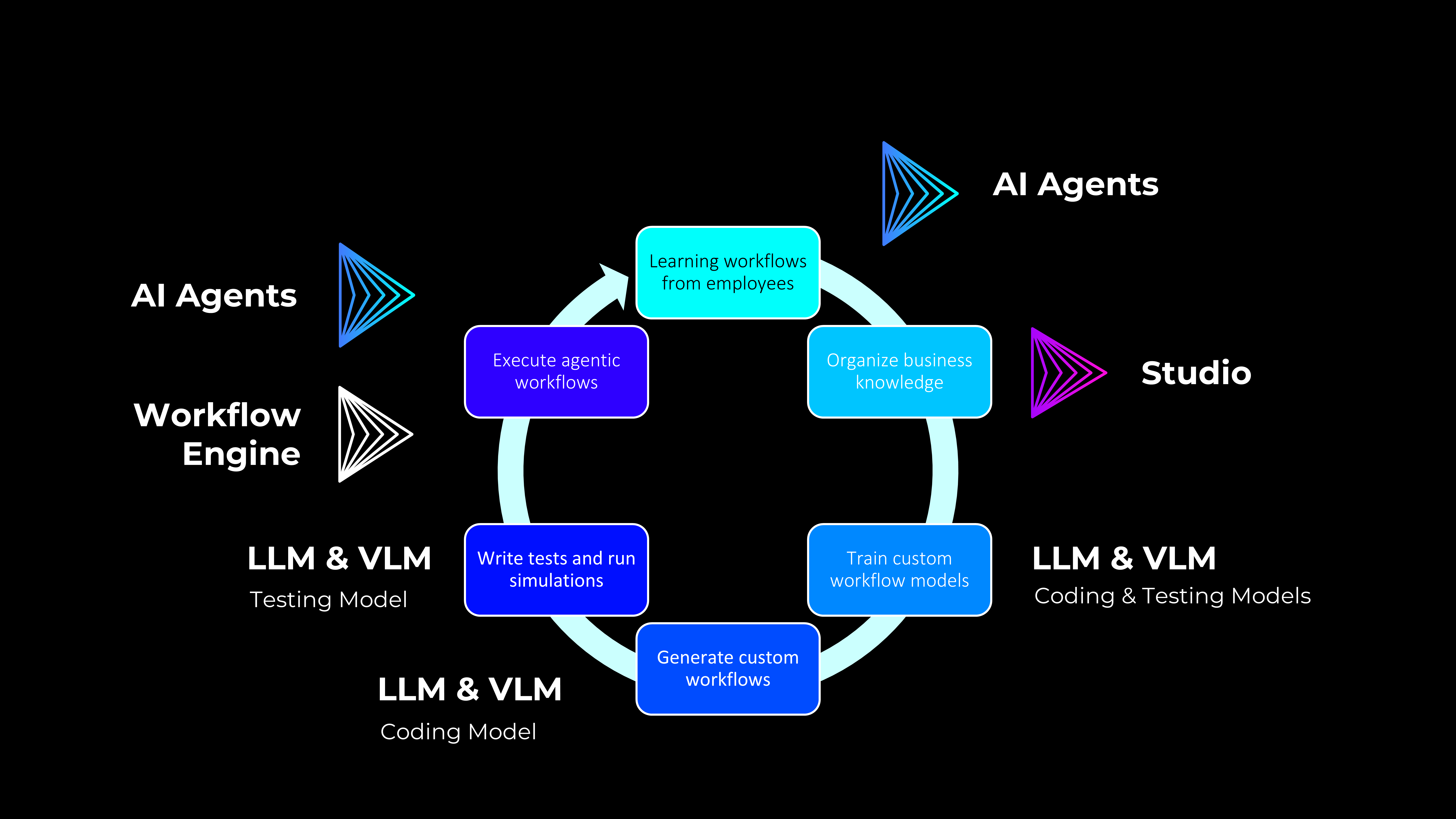
It starts with our premier AI agent for browser automation called Browser Agent that, given a command in natural language, executes it by controlling a Chromium-based web browser. The platform continues with two more AI agents – OpenAPI Agent that makes it easy to blend in calls to existing systems via REST APIs, and Prompt Agent that performs calls to different large language models to solve different intelligence tasks that don’t require immediate interaction with web or desktop apps or web services. More AI agents, like Desktop Agent and Document Agent, are in the works, to complement our initial AI agents in our goal to transform organizations into agentic ones.
The platform further expands by making a Studio application that makes it possible to design, test, edit, deploy, and monitor robust automations in both test and production environments. The platform completes the full process with Workflow Engine that runs these automations at the organizational scale, with infrastructure for running web and beyond web automations inside the secure perimeter.
We are super excited to bring Sentius Teach & Repeat Platform to life – a platform designed from the ground up to help organizations become agentic by reliably performing complex tasks using Sentius AI agents.
First Release
There are two kinds of tasks that companies want to automate: known and unknown. For example, when a private aviation company wants to book slots for its airplanes in each airport, the website itself is known as well as the task. But when a public pension fund wants to download quarterly financial presentations of public companies, the number of companies is too big, and thus the way to solve tasks differs from one company’s website to another. The only way to create automation to interact with known websites was robotic process automation, but such automation always breaks down due to the ever-changing nature of the web. And there was no way to create automation to interact with unknown websites at all. Until now.
Sentius Browser Agent uses the power of combining both analytical and model-free methods (rule-based and neural network-based) approaches to solve both kinds of tasks. Like humans, it can learn from others how to perform a particular task by imitation, and it can learn on the go while trying to solve a previously unknown task on its own. A successful task can then be saved as an instruction, and, like humans apply learnt skills in their everyday lives, Sentius Browser Agent can re-use known instructions to execute known tasks. And if the problem arises, it can leverage its ability to find new solutions and deliver expected outcome.
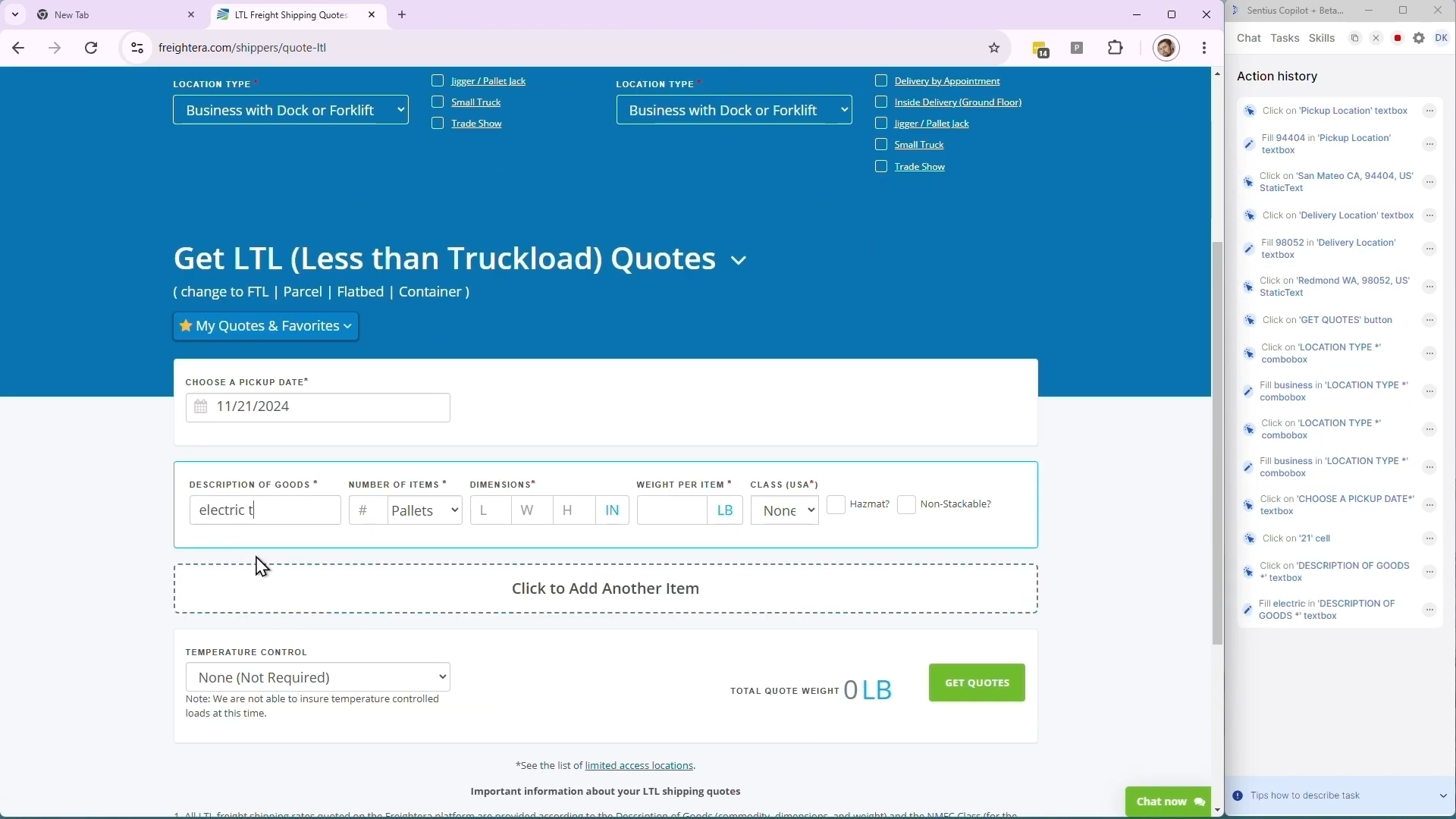
To ensure that given tasks can be easily integrated together with calls to existing services via APIs, Sentius Studio makes it easy to compose workflows that combine instructions for both Browser Agent and OpenAPI Agent together.
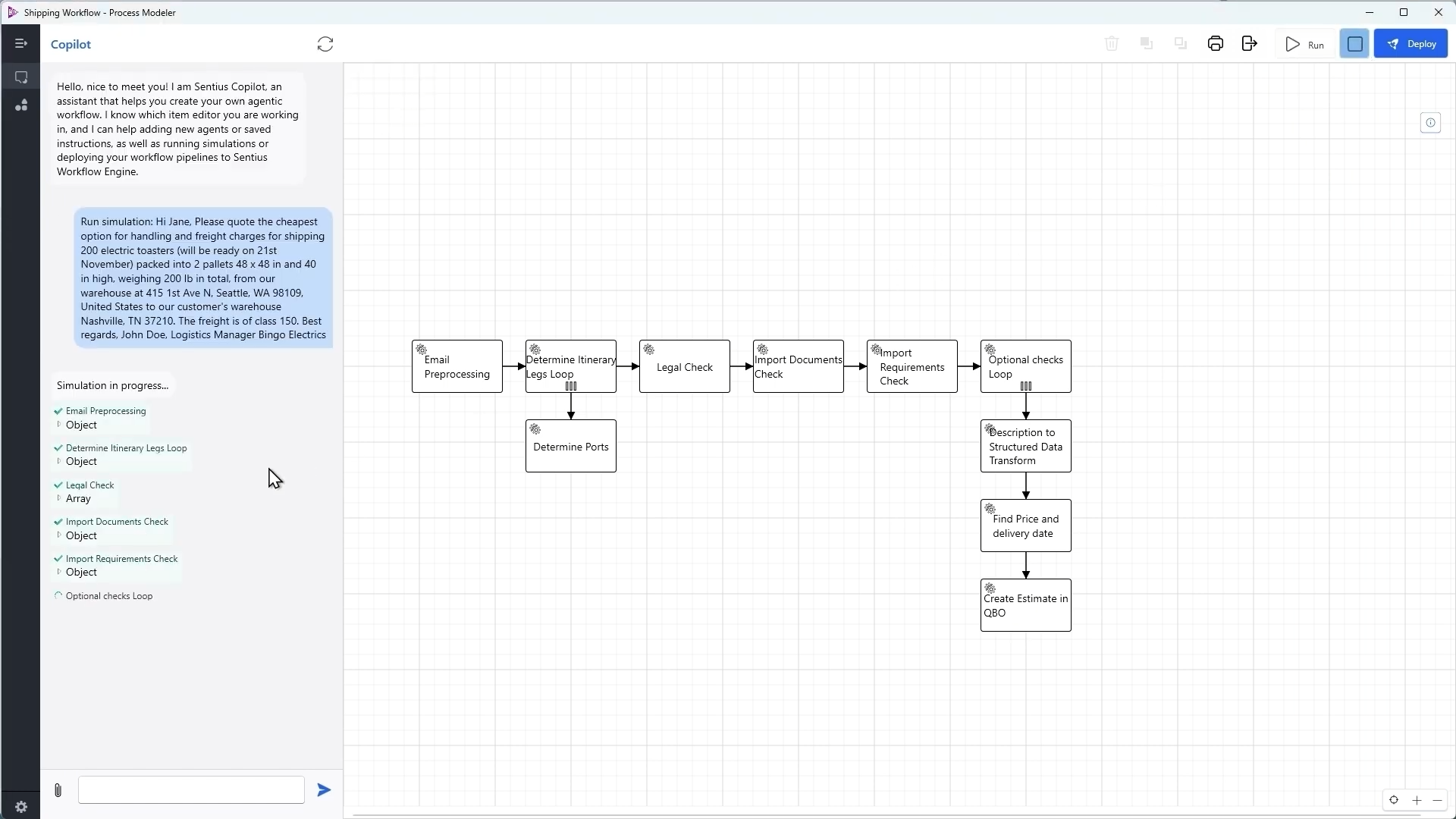
The private beta that is already used by our design partners and customers, includes:
- Sentius Browser Agent available via Sentius Copilot+ app for Windows, MacOS, and Ubuntu, that can be interacted with directly via chat interface and via API,
- Sentius Studio as an app currently available for Windows (and later to be available for MacOS), built to create, test, deploy, and monitor complex workflow automations involving Browser Agent, OpenAPI Agent, Prompt Agent, humans via human-in-the-loop, and more agents (Desktop, Document etc.) coming in 2025 and beyond,
- Sentius Workflow Engine as a service to run complex automations at organizational scale, also available via APIs.
Reliable Agents at Your Fingertips
Browser Automation is inherently a complex task: while it can be very simple like opening a search engine web page, entering text, and clicking on the first search result followed by retrieval of the contents of that first search result, it is impossible to anticipate all possible tasks given to a Browser Agent to automate.
To make Sentius Browser Agent reliable at its core, we have enabled it with the ability to learn successful paths for solving tasks, and then re-using them in further task executions, ensuring reliability sought by organizations. Excellence of our browser agent is shown by its performance on WebVoyager benchmark.
But that’s just the beginning. As an organization coming from the applied and fundamental research roots, we are looking beyond what is available today by working on hybrid methods aimed at making underlying foundational models exceptional in performing structured problem-solving. These methods, like Associative Recurrent Memory Transformer recognized at ICML, are the best in the world in enabling language models today to do reasoning at long context scale. Their state-of-the-art performance on the BABILong benchmark was recently spotlighted at NeurIPS 2024.
To learn more about the technology behind Sentius Teach & Repeat Platform, click here.
From First Release to Making Agentic Enterprise
Our vision is to move from the tool for to designing, testing, deploying, and monitoring workflows to the tool designed to for controlling all aspects of the agentic enterprise. We see how combination of visual tools, AI agents powered by hybrid foundational models, workflow engine, and complex long-term memory systems powered by knowledge graphs and vector databases, will become a new brainpower of the agentic organizations.
We will continue our work in several directions:
- Making our Browser Agent even more reliable and cost-effective by using customized foundational models,
- Making our Sentius Studio even simpler to test, deploy, and monitor automations, and then expand it towards managing the entire agentic enterprise,
- Making our Workflow Engine capable of automatic infrastructure scaling to address the process of transforming organizations of our customers into the agentic form,
- Adding more Agents like Desktop and Document (but not limited to them) to cover more areas of workflow automations,
- Building the next generation of Foundation Agentic Models on top of the ARMT technology to power the reliable and cost-effective AI agents of tomorrow.
At Sentius, we believe that the path towards agentic enterprise needs both applied and fundamental research, but it starts with tooling that helps to automate first workflows today. Most of the systems used by organizations involved in existing processes are either web-based or API-based, and our initial release is focused on combining them together to make the first step towards our vision.
Each time we automate repeatable, tedious, boring tasks, we make it possible for those who used to perform them, open to more strategic tasks that require human input.
At Sentius, we are super excited about the future of human civilization powered by agentic organizations, and our goal is to lead this transition together with our design partners, customers, investors, and friends.
Join us in making organizations agentic - click here.